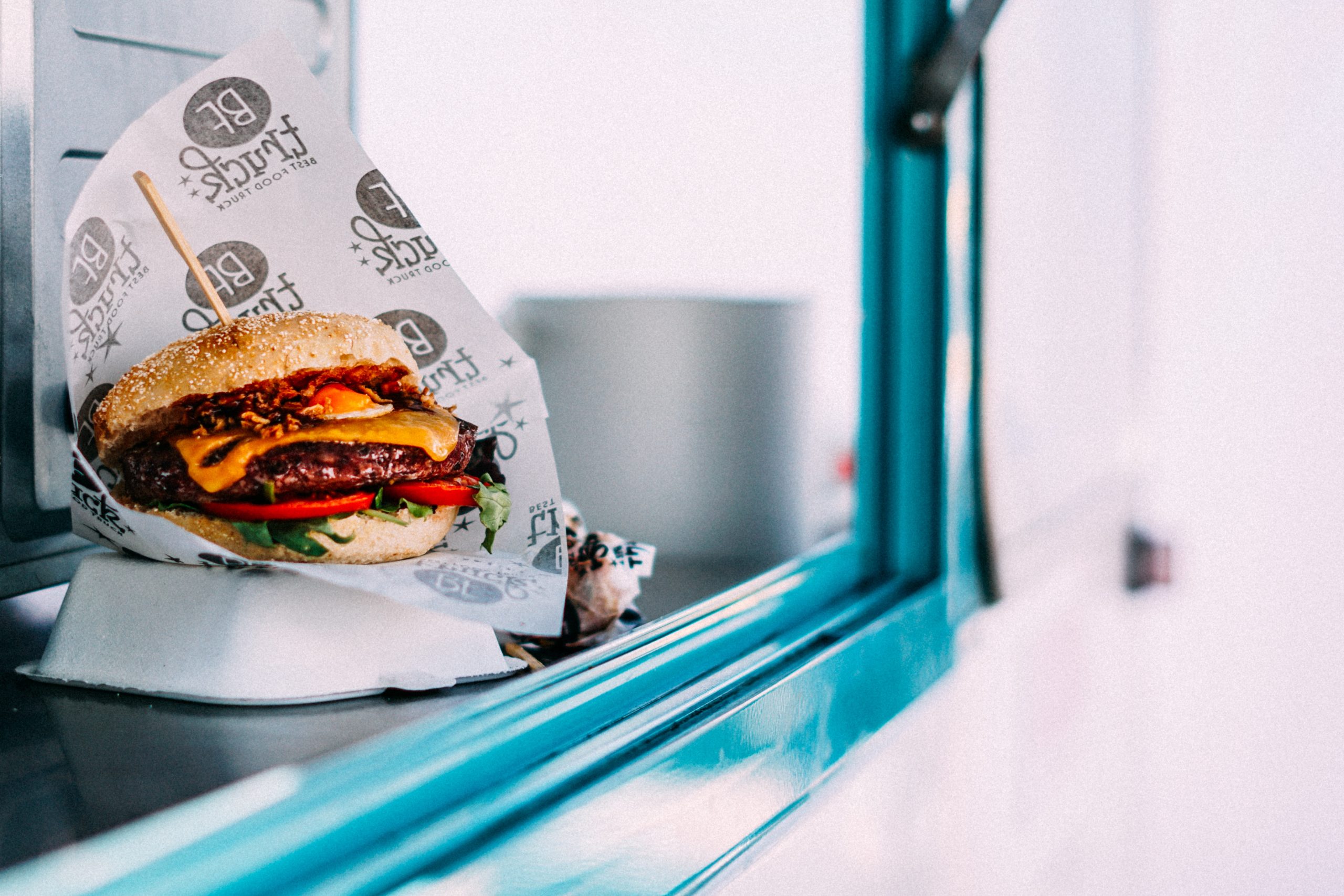
Setting Prices in Context
Authors: Krishna Arangode, Anand Srinivasan
The Impact of Pricing in Context
Incorporating the context of your customer’s purchase decision into your pricing analytics unlocks opportunities that are hidden at a segment level, and has yielded an additional 1% to 2% revenue uplift over and above a company’s existing Pricing and Revenue Management capabilities – at times exceeding a 10% revenue increase on a specific transaction. These results can be the difference between surprising The Street with your outperformance and struggling to explain the challenges you faced in underperforming during the prior quarter.
Setting Prices in Context
Although Revenue Management (RM) has historically been built with the objective of identifying and quantifying the context of a customer’s purchase decision, several constraints have resulted in the contexts being generated at a macro-level – such as days prior, length of stay, origin/destination pair, day of week, etc. Two of these constraints are the inability to incorporate unstructured data into traditional statistical methods, and the inability to process a large amount of data in a timely manner to generate price recommendations while they are still relevant to the context. Understanding the true context behind a decision is certainly not a new concept; rather, it has historically been conducted as marketing studies using methodologies such as purchase funnel analysis. However, these contexts have not been factored into the analytical solution that determines the right price for the company’s products/services.
But with the rapid growth in data acquisition technologies and the explosion of self-service data management tools, we have been able to take RM to the next level of performance and granularity by incorporating the context of a customer’s purchase decision into a company’s pricing decisions. We have created and leveraged a Contextual Pricing Framework (CPF) in several industries and business models to incorporate context into RM applications – and we have done this analytically, rapidly, and with significant value derived.
Examples of these applications are described in greater detail in this POV.
Examples of Setting Prices in Context
In the table below, we provide several real-world examples of companies who have identified opportunities and captured incremental revenue uplift by incorporating more focus on the context of a customer’s purchase decision, leveraging a CPF within their RM application.
In each of the above examples, a shift in context for a specific customer purchase decision creates new opportunities for a company to use price as a lever to expand margins. Every shift in context must be measured and translated into a price premium/discount to be applied when the context occurs. The estimation of the premium/discount in each context is driven by a combination of art and science, incorporating a framework, new processes, data and tools.
Deploying a Contextual Pricing Framework
Our toolkit of Kaizen ValueAccelerators™ has been leveraged to rapidly design, develop, and deploy contextual pricing frameworks. Working from customer data supplied by our clients, our toolkit brings proprietary metrics and publicly-available data sources to quickly generate a “business-reasonable” starting point for making pricing decisions in a more relevant context. This allows us to provide our clients with unique, “out-of-the-box” insights on price premiums/discounts for each context.
- Stage 1 / Establish Hypotheses: The Kaizen and client business subject matter experts (SMEs) establish a list of all possible situations that define context for the client’s customer transactions. The outcome of the first stage is a succinct list of hypotheses for testing, along with the associated criteria that either prove or disprove each hypothesis.
- Stage 2 / Test Hypotheses: The Kaizen and client analytics/data science teams gather the data needed and test hypotheses against the success criteria to prove or disprove each hypothesis. The outcome of the second stage is a list of proven hypotheses that clearly define the context(s) for the client.
- Stage 3 / Configure KVAs (Kaizen ValueAcceleratorsTM): The Kaizen and client analytics/data science teams partner in configuration of existing KVAs and development of any additional analytical models, with the goal of capturing the value associated with each context and the associated pricing opportunity. The outcome of the third stage is either an automated process for context value capture and a price recommendation engine, or a one-time snapshot of potential price recommendations for specific contexts.
- Stage 4 / Model Testing & Refinement: The Kaizen team tests the models developed against a variety of situations to gather feedback on model performance with different business dynamics at play. The outcome of the fourth stage is a set of models that: (1) are refined based on testing results; and (2) generate the final market-ready set of context-informed price recommendations.
- Stage 5 / Market Test Prices: The Kaizen and client SMEs identify a small pilot “market” in which the context-informed recommendations can be implemented. The recommended prices are put into effect in a controlled setting with close observation on impacts from the joint Kaizen and client teams. During this test period, refinements of the models and recommendations are continually worked based on the feedback provided and observed. It is important during this phase to isolate the effect of prices versus market dynamics to avoid over-correcting the models.
- Stage 6 / Implement Prices: Upon successful completion of the pilot, the models and pricing strategies developed are deployed across the client business line. The field operators are trained on the new pricing strategies and models are continually refined by the client analytics/data science team.
Key Context Enablers: Data & Tools/Technology
The CPF approach requires data on a series of attributes associated with the transaction, including price, volume, time dimensions (such as day of week/month/time of day, etc.), customer attributes, channel, surrounding factors (such as other transactions at the same/surrounding time windows, etc.). In addition to a company’s internal data, we have also leveraged cleansed and transformed data from publicly-available data sources to boost model results, if needed.
Today, there are scores of analytical tools and techniques used as part of a CPF approach, from supervised learnings to unsupervised learning approaches to a combination of both. Some of the common tools utilized include Random Forest, Classification and Regression Trees, multivariate regressions, and clustering techniques such as K-means. In our experience providing analytics services across multiple industries, any analytics deployment must balance mathematical accuracy of the underlying models with the business rules that dictate the real-world operational constraints faced by clients.
A classic example of this balance is the operational differences between ticketing items (physically affixing prices to goods, such as merchandise in a retail outlet) and color-coded or digital signage. The pricing recommended by CPF may drive frequent price changes. These price changes can likely be supported quite easily for companies that employ color-coded pricing or digital signage. For companies who must ticket their inventory, re-ticketing to support frequent price changes is an unrealistic operational process. In this scenario, the results from CPF should be passed through an evaluation consisting of a cost-benefit comparing the cost of re-ticketing inventory versus the expected uplift from new prices. New prices go into effect only when the benefits significantly outweigh the costs. Such business rules sit on top of every tool that is developed as a part of the CPF.
Summary
A Contextual Pricing Framework allows you to incorporate the context of your customer’s purchase decisions into your company’s pricing analytics. This approach:
- Determines the true value that your customer associates with your products/services within a given context
- Unlocks pricing opportunities that are hidden at a segment or other more aggregate level
- Yields an additional 1% to 2% revenue uplift over and above existing Pricing / Revenue Management capabilities
Kaizen Analytix’ toolkit of Kaizen ValueAccelerators™ has been used to rapidly employ contextual pricing. Working from customer data supplied by our clients, our toolkit brings proprietary metrics and publicly-available data sources to quickly reach a “business-reasonable” starting point for making pricing decisions in a more relevant context.
More Publications
-
Automotive Innovation Series, Part 4: Harnessing Unsupervised Machine Learning in the Automotive Sector
-
The Future of Payment Infrastructure: Overcoming Challenges & Embracing Innovation
-
The Current State of the Financial Services Industry: Key Challenges & Priorities for the Future
-
The Current State of Credit Unions: Challenges, Trends, and Solutions for Sustainable Growth