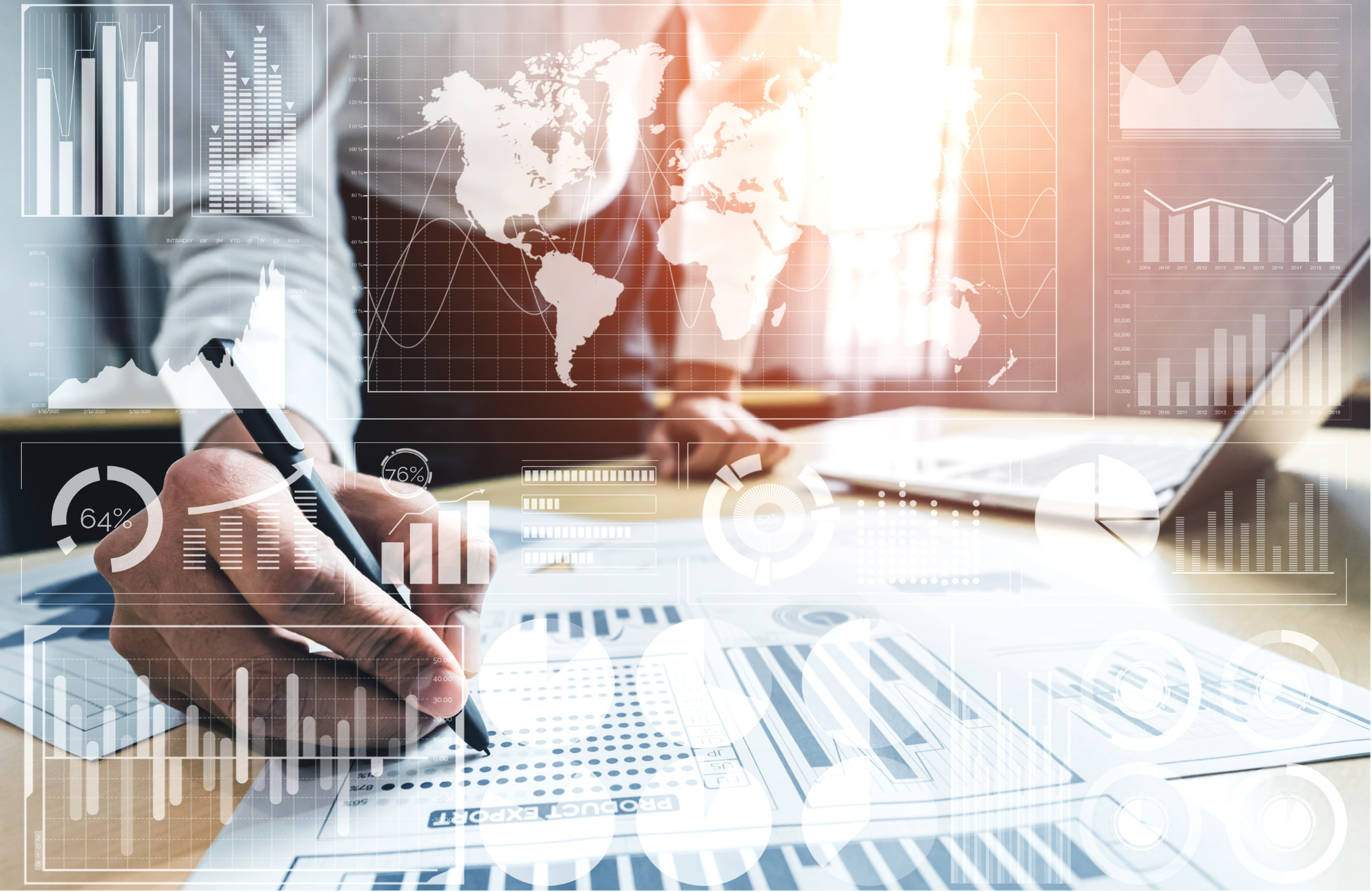
Point of View: Strengthening Data Quality & Governance Is a Strategic Imperative for Asset and Wealth Management Firms
Authors: Nagendra Sherman, Kaizen Analytix, LLC
Introduction
In today’s fast-moving, digitally-driven financial landscape, data is more than a byproduct of operations – it’s the lifeblood of strategic decision-making. Nowhere is this more evident than in asset and wealth management, where every function – from portfolio construction to client experience to compliance – is fueled by data.
Many firms continue to struggle with foundational issues around data quality and governance. Despite heavy investments in analytics and digital platforms, the benefits often remain limited by fragmented systems, inconsistent data standards, and manual, error-prone processes.
Fixing this limitation isn’t about checking a regulatory box or running a one-time data cleanup. It’s about building lasting confidence in your data—confidence that underpins every decision, interaction, and innovation. And in an industry where trust is the ultimate currency, that confidence can’t be overstated.
The Expanding Role of Data in Wealth and Asset Management
Asset and wealth management firms rely on a vast and diverse ecosystem of data: market feeds, custodial transactions, client portfolios, performance benchmarks, financial statements, third-party research, and more. This data supports investment strategies, powers AI-driven insights, enables client reporting, and keeps the firm in regulatory compliance.
But when data is siloed, outdated, or of poor quality, it creates serious downstream consequences. These include:
- Inaccurate or delayed client reporting
- Operational bottlenecks and rework
- Suboptimal investment decisions
- Missed cross-sell or upsell opportunities
- Regulatory risk and reputational damage
In contrast, firms with trusted data can move faster, serve clients better, and scale more efficiently. They create more meaningful digital experiences and are better prepared to embed advanced analytics and AI into their operations.
What Makes Asset and Wealth Management Unique
Compared to other financial verticals, asset and wealth managers face a distinct combination of data challenges:
- High Volume and Variety: Firms ingest structured and unstructured data from custodians, clients, research partners, and internal systems. Integrating these sources seamlessly is often difficult.
- Legacy Infrastructure: Many firms still operate on decades-old platforms that don’t enforce consistent standards or integrate well with modern systems.
- Disparate Business Units: Wealth management, institutional asset management, and alternatives may each have their own data systems and governance rules, creating duplication and inconsistency.
- Manual Workarounds: Many workflows still rely on spreadsheets, emails, and offline approvals, slowing down processes and increasing the potential for human error.
The cumulative effect? Data that is difficult to trust, and even harder to leverage strategically.
A Framework for Modernizing Data Governance and Quality
Solving this challenge requires a strategic, enterprise-wide approach. Here are six key pillars that leading firms are adopting:
- Define Clear Data Ownership and Accountability
Assign data stewards at both the enterprise and business-unit levels. These roles are responsible for maintaining data accuracy, completeness, and compliance, ensuring accountability across the data lifecycle. - Develop an Enterprise Data Governance Policy
Establish a central policy that outlines shared definitions, lineage standards, access protocols, and stewardship models. The framework should be flexible enough to adapt to the distinct needs of different business lines but unified enough to eliminate duplication and inefficiency. - Invest in AI-Enabled Data Quality Tools
Use intelligent tools to detect anomalies, reconcile inconsistencies, and clean data in real time. Real-time dashboards can help visualize data health metrics and drive proactive remediation, while learning algorithms adapt to evolving patterns over time. - Embed Governance into Every Digital Initiative
Data governance isn’t something you apply after a platform is built. It must be embedded from the start—into cloud migrations, analytics implementations, new product launches, and client-facing platforms. - Build a Data-Driven Culture Across the Organization
Successful governance requires buy-in from every level. Offer training, celebrate good data practices, and promote cross-functional collaboration. The goal is to make data quality everyone’s job—not just IT’s responsibility. - Enable Secure, Real-Time Access Across Teams
Implement architectures such as data fabric or data mesh to support distributed access to a shared data layer. This allows different teams to self-serve data while maintaining strict controls over security, quality, and compliance.
The Shift to Centralized, Intelligent Data Platforms
Many firms are moving away from fragmented, tool-based approaches toward centralized, intelligent data management platforms. These platforms integrate ingestion, transformation, quality assurance, access control, and lineage tracking into a single ecosystem—delivering consistency, automation, and visibility across the enterprise.
What sets these solutions apart is their ability to use AI not only to cleanse data, but to continuously learn and improve it over time. They automate quality checks, surface potential risks before they become issues, and offer a unified view of the firm’s data landscape. They also scale easily across business lines, adapting to both current and future needs.
The Business Case for Trusted Data
Firms that consistently invest in data quality and governance see benefits across four key dimensions:
- Faster, Smarter Decisions: Better data accelerates time-to-insight and improves confidence in investment decisions.
- Enhanced Client Experience: Accurate reporting and personalized insights build trust and satisfaction.
- Operational Efficiency: Automation and standardization reduce manual work and free up staff to focus on higher-value tasks.
- Regulatory Confidence: Auditable data processes and transparent lineage reduce the risk of non-compliance and increase preparedness for exams.
Conclusion
In asset and wealth management, data is no longer just an enabler—it’s a differentiator. But data is only as valuable as it is trustworthy. Strengthening data quality and governance is not a cost center but rather a strategic advantage.
Firms that get this right will comply more easily and operate more efficiently. Even more, they’ll build stronger client relationships, unlock more value from AI and analytics, and position themselves for long-term success in a rapidly evolving industry.
More Publications
-
Automotive Innovation Series, Part 4: Harnessing Unsupervised Machine Learning in the Automotive Sector
-
The Future of Payment Infrastructure: Overcoming Challenges & Embracing Innovation
-
The Current State of the Financial Services Industry: Key Challenges & Priorities for the Future
-
The Current State of Credit Unions: Challenges, Trends, and Solutions for Sustainable Growth