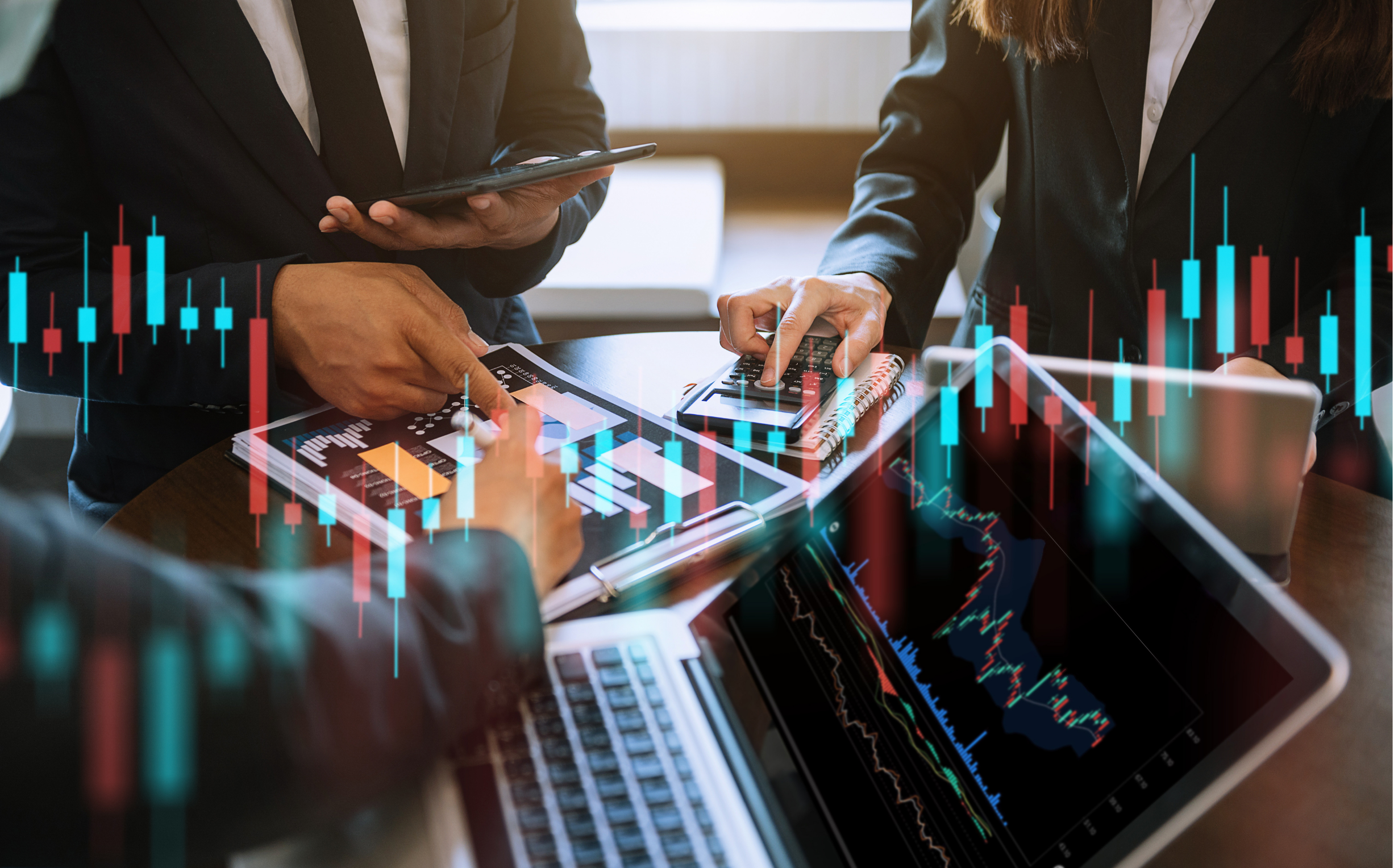
Driving Value Through Smarter Fee Structures in Asset Management
Authors: Nagendra Sherman, Kaizen Analytix, LLC
Introduction
The asset management industry is undergoing a profound shift. Faced with intensifying competition from low-cost passive funds, the rise of fintech disruptors, evolving client expectations, and increased regulatory oversight, traditional pricing models are becoming increasingly unsustainable. Clients are no longer content with standardized, opaque fee structures. They expect pricing that is transparent, performance-linked, and tailored to the value they receive.
As a result, many asset management firms are re-evaluating their fee strategies, seeking more agile, client-centric approaches that balance profitability with trust. One of the most powerful enablers of this shift is the integration of predictive analytics and real-time benchmarking. These advanced tools allow firms to move beyond static pricing frameworks and embrace a dynamic, data-driven model—one that personalizes fee structures, strengthens client relationships, and provides a sustainable competitive edge.
The Limitations of Traditional Fee Structures
Historically, fee structures in asset management have been relatively straightforward: most commonly a flat percentage based on assets under management (AUM). While this model offered simplicity and predictability, it often failed to reflect either the nuanced needs of different client segments or the actual value delivered by the firm.
Today’s clients, particularly high-net-worth individuals and institutional investors, are more informed and value-conscious. They expect fee arrangements that reflect performance, service levels, and the complexity of their portfolios. At the same time, global regulatory bodies are increasing pressure on firms to clearly disclose fees and justify their pricing with measurable outcomes.
Traditional models also tend to rely on outdated systems and manual processes that limit agility. Without access to real-time insights, many firms are unable to analyze pricing effectiveness, benchmark against peers, or adjust their structures in response to market shifts. This creates a significant risk of client dissatisfaction, revenue leakage, and competitive erosion.
The Case for Data-Driven Fee Optimization
To remain competitive in this environment, asset management firms must adopt a more strategic and responsive approach to pricing. This is where predictive analytics and real-time benchmarking come into play.
Predictive analytics uses historical data, client behavior, and market trends to forecast outcomes and inform pricing decisions. It allows firms to anticipate client needs, evaluate pricing strategies across segments, and proactively manage profitability. By understanding how different client types respond to various fee models, firms can design pricing strategies that resonate more deeply and deliver higher value.
Real-time benchmarking enables firms to compare their fee structures to those of their competitors and to prevailing industry norms. This ensures that pricing remains competitive and aligned with client expectations. Benchmarking also helps firms identify trends, such as the rise of performance-based or subscription models, so they can adapt before falling behind.
Together, these capabilities form the foundation for personalized pricing strategies that reflect both the value delivered and the specific preferences of each client segment.
Building Personalized Pricing Models
The real power of predictive analytics and benchmarking lies in their ability to support personalized fee structures—flexible pricing models that align with different client needs, behaviors, and investment goals. Examples of such models include:
- Tiered AUM Fees: Scaling fee percentages based on asset size, rewarding clients who consolidate assets with more favorable pricing.
- Performance-Based Fees: Linking fees to fund performance or portfolio outcomes, aligning firm incentives with client success.
- Subscription-Based Models: Offering flat-rate pricing for certain services, providing cost predictability and appealing to younger, digitally savvy clients.
- Hybrid Models: Combining elements of AUM, performance, and flat fees to suit more complex client requirements.
These models not only address the demand for personalization but also provide firms with greater flexibility to respond to market volatility, product performance, and evolving client expectations.
Strategic Benefits for Asset Management Firms
Firms that integrate predictive analytics and benchmarking into their pricing strategy can unlock a wide range of business benefits:
- Enhanced Client Trust and Loyalty
Transparent, personalized fee structures demonstrate a commitment to fairness and value, deepening client relationships and boosting retention. - Improved Client Acquisition
Offering flexible and innovative pricing models sets firms apart in a crowded market, attracting new clients—especially those looking for alignment between fees and outcomes. - Increased Revenue and AUM
By identifying underperforming pricing strategies and correcting them proactively, firms can optimize revenue per client. Satisfied clients are also more likely to consolidate assets under management. - Greater Operational Efficiency
Automating fee calculations and compliance reporting reduces administrative burden, allowing teams to focus on higher-value tasks and improving cost efficiency. - Reduced Regulatory Risk
Clear, auditable pricing structures and automated disclosures enhance compliance and minimize the likelihood of regulatory penalties or client disputes. - Sustainable Competitive Advantage
The ability to adapt pricing in real time—based on performance, market trends, and peer comparisons—ensures that firms remain agile and relevant in a fast-evolving industry.
Conclusion
In today’s client-centric, regulation-heavy, and increasingly competitive market, optimizing fee structures is no longer optional—it’s essential. Asset management firms must shift from traditional, static pricing to a more dynamic and data-driven model that reflects value, builds trust, and fuels long-term growth.
Predictive analytics and real-time benchmarking provide the insights and flexibility needed to personalize pricing, meet evolving client expectations, and maintain a strong market position. Those who invest in these capabilities now will be best positioned to lead the next chapter of innovation in the asset management industry.
More Publications
-
Automotive Innovation Series, Part 4: Harnessing Unsupervised Machine Learning in the Automotive Sector
-
The Future of Payment Infrastructure: Overcoming Challenges & Embracing Innovation
-
The Current State of the Financial Services Industry: Key Challenges & Priorities for the Future
-
The Current State of Credit Unions: Challenges, Trends, and Solutions for Sustainable Growth