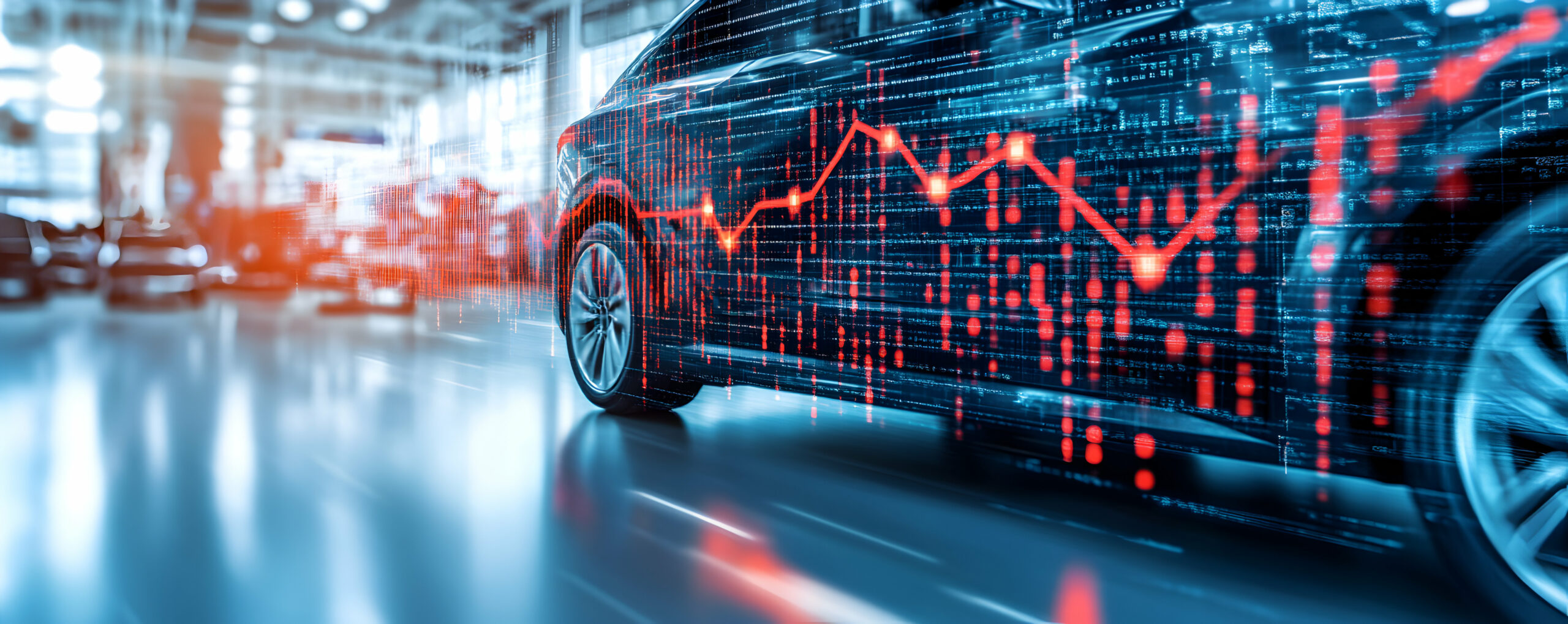
Automotive Innovation Series, Part 3: Forecasting Using ML & Data Science
Authors: Kishore Neppalli, Kaizen Analytix, LLC
Introduction
In the ever-evolving automotive industry, accurate forecasting is not just beneficial; it’s essential for driving innovation and maintaining competitive advantage. With rapid changes in consumer preferences, supply chain dynamics, and technological advancements, manufacturers must harness the power of data to make informed decisions. At Kaizen, we employ sophisticated forecasting models that leverage machine learning (ML) and data science to empower automotive organizations to anticipate market trends and optimize their operations.
In this post, we delve into key forecasting techniques that are transforming the automotive sector.
Key Forecasting Models and Techniques:
- Extensive Forecast Library:
- A robust forecasting library is crucial for any organization looking to enhance its predictive capabilities. At Kaizen, we leverage an extensive library of custom-tailored forecasting models designed to a variety of forecasting problems in the automotive industry. This library includes not only standard set of forecasting techniques such as time series analysis, causal models, and regression-based approaches, but also augmented with advanced techniques such as feature engineering, numerical transformation, assembling augmented with our Kaizen automotive business expertise. This enables us to select the most suitable model based on the specific data characteristics and forecasting requirements. By utilizing an extensive forecast library, we ensure that our clients can generate reliable forecasts that align with their strategic objectives.
- Pick-Best Methodology:
- The pick-best methodology is a crucial step when designing innovative forecasting solutions that involves evaluating multiple models to determine which one yields the most accurate predictions for a given dataset. This technique allows organizations to capitalize on the strengths of various models, selecting the best-performing one based on historical data. By employing the pick-best methodology, automotive companies can achieve greater accuracy in their forecasts, enabling them to optimize inventory levels, manage production schedules, and respond proactively to market demands.
- Forecast Accuracy Measurement:
- Measuring forecast accuracy is vital for refining forecasting models and enhancing their reliability. At Kaizen, we implement various metrics to assess forecast accuracy, such as Mean Absolute Percentage Error (MAPE), Root Mean Squared Error (RMSE), and Mean Absolute Error (MAE). By continuously monitoring and analyzing these metrics, we can identify areas for improvement and make necessary adjustments to our forecasting models, ensuring that they remain aligned with actual outcomes.
- Forecast Accuracy Expectation Setting:
- Setting realistic expectations for forecast accuracy is a critical aspect of the forecasting process. It is essential for organizations to understand the inherent uncertainties in their data and the limitations of their forecasting models. By establishing clear expectations for forecast accuracy, automotive companies can better align their operational strategies and decision-making processes with the realities of market conditions. This proactive approach not only fosters confidence in forecasting outcomes but also enables organizations to plan effectively for various scenarios.
Conclusion
As the automotive industry continues to embrace digital transformation, the importance of accurate forecasting cannot be overstated. By leveraging advanced forecasting models and methodologies, organizations can enhance their ability to anticipate market trends, optimize operations, and drive innovation. At Kaizen Analytix, we are committed to helping our clients harness the power of ML and Data Science to develop robust forecasting capabilities that fuel their growth and success.
Stay tuned for the next installment in our Automotive Innovation Series, where we will explore the role of Unsupervised Machine Learning in enhancing operational efficiency and decision-making in the automotive sector. Plus, don’t miss our Automotive Series Part 1 on Statistical Analysis and Part 2 on Optimization!
More Publications
-
Automotive Innovation Series, Part 4: Harnessing Unsupervised Machine Learning in the Automotive Sector
-
The Future of Payment Infrastructure: Overcoming Challenges & Embracing Innovation
-
The Current State of the Financial Services Industry: Key Challenges & Priorities for the Future
-
The Current State of Credit Unions: Challenges, Trends, and Solutions for Sustainable Growth