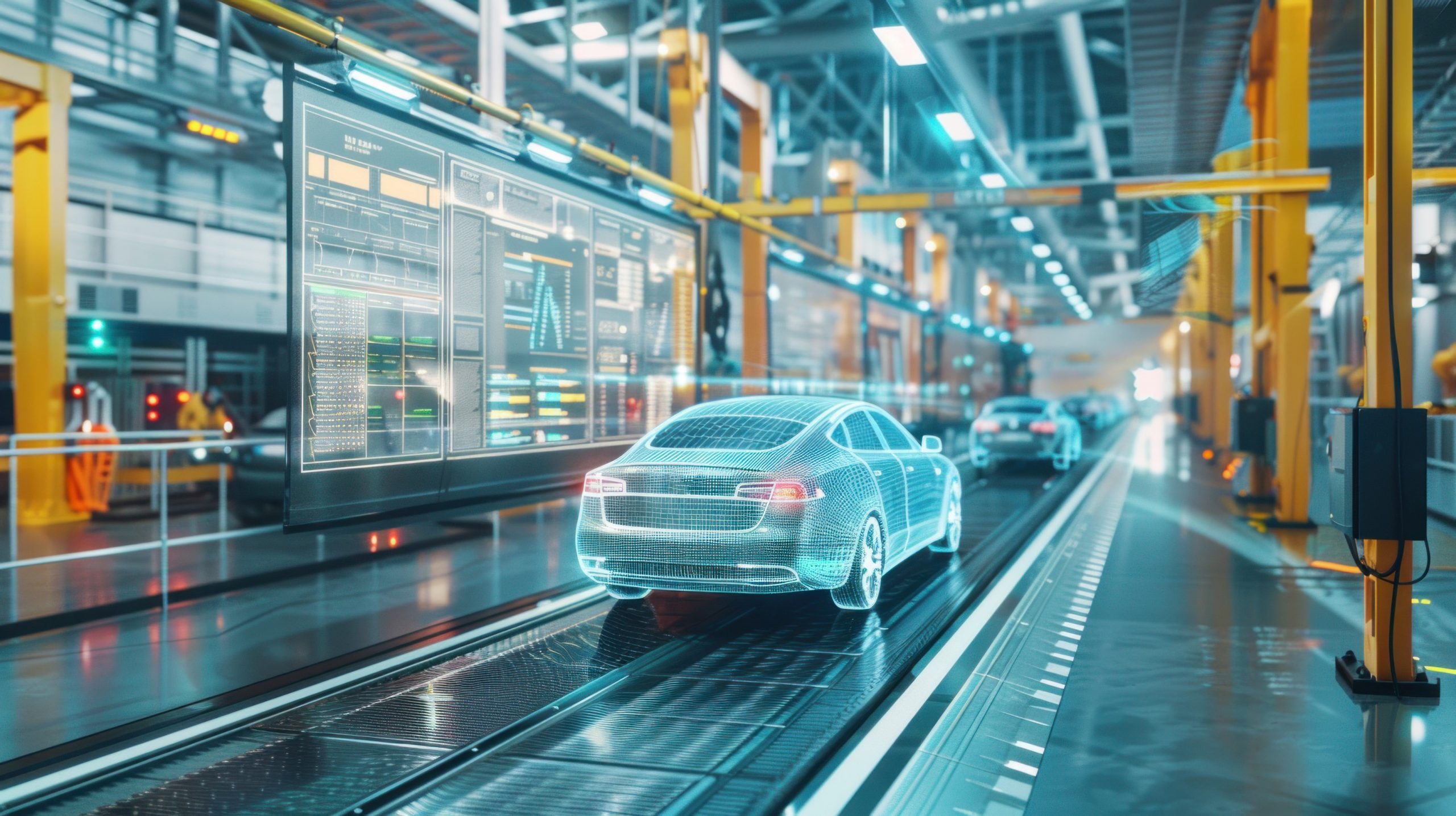
Automotive Innovation Series, Part 1: Statistical Analysis with AI, ML, and Data Science
Authors: Kishore Neppalli & Krishna Arangode, Kaizen Analytix, LLC
Introduction
In the automotive industry, innovation isn’t just a buzzword; it’s the very engine that drives growth, efficiency, and sustainability. From the drawing boards of designers to the assembly lines of manufacturers and the operational strategies of suppliers, distributors, and dealers, every facet of the automotive sector is undergoing a significant transformation, thanks to the advent and integration of Artificial Intelligence (AI), Machine Learning (ML), and Data Science.
At Kaizen, we have over a decade of experience as “trusted partners” of senior management professionals and decision-makers in the automotive industry. While GenAI tools like ChatGPT and Google Gemini have gained tremendous popularity – for us at Kaizen, we’ve been using AI/ML in several of our engagements for a few years now.
We combine deep experience in Artificial Intelligence, Machine Learning, and Advanced Data Science with a focus on the business context. Our data scientists along with Automotive SMEs develop custom tailored models that power the software, products, advanced analytics, and AI services we provide. We thrive not only for accurate and efficient models, but also for highly explainable aspects that could take it one step further in forecast reasoning.
Within the realm of Data Science, Kaizen offers deep expertise in the below mentioned areas which are applied to the wide spectrum of the client’s interest including, incentive recommendation engines, marketing strategizing, pricing engines, manufacturing process optimization/streamlining:
- Statistical Analysis
- ML-powered Optimization
- Forecasting
- Supervised and Unsupervised Machine Learning
- ML-powered Price Modeling
- Prescriptive Analytics
Decision Velocity Matters in the Automotive Sector
Our data science models – along with subject-matter experts and domain specialists – make life easier for senior-level decision-makers. They help go from “data to insight to action” as quickly as possible. In short, we help increase decision velocity – which is essentially better decisions, faster decisions – that propel companies forward in the right direction.
To explain our data science capabilities, Kaizen Marketing is now creating a series of blogs that give our readers a sneak peek into what lies under the hood. We’re planning a series of six posts in each of the aforementioned areas.
The automotive industry, with its intricate supply chains, strict safety standards and evolving consumer preferences, leverages AI, ML, and Data Science to tackle challenges and explore new opportunities. Whether it’s through enhancing vehicle design and performance with predictive analytics for incentive planning, vehicle pricing and profit optimization; streamlining manufacturing processes with intelligent automation leveraging sophisticated application development practices, or revolutionizing customer experiences with personalized digital experiences, these technologies are at the forefront of the industry’s evolution.
Our first blog in this series will focus on the application of statistical analysis in the automotive industry.
Usage of Statistical Analysis in the Automotive Sector
Statistical analysis plays a crucial role in the automotive industry, providing insights that help manufacturers and stakeholders make informed decisions, improve products, and optimize processes.
Let’s explore how the specific statistical concepts can be applied in this sector:
Descriptive / Multivariate Statistics
- Descriptive statistics help summarize and understand the basic features of a dataset, such as the mean, median, mode, and standard deviation. In the automotive industry, this can be applied to analyze customer satisfaction surveys, vehicle performance metrics, and production data to understand current trends and performance levels.
- Multivariate statistics are used when multiple variables are involved, and the goal is to understand the relationships between them. This can be applied in the automotive sector for (just a few examples):
- Market segmentation: Identifying different customer groups based on their preferences, behaviors, and demographic factors.
- Vehicle Design optimization: Analyzing how various design parameters (such as weight, engine power, and fuel efficiency) interact and affect the overall vehicle performance.
Regression, Predictive, Cohort and Variance Analysis
- Regression and predictive analysis are used to predict outcomes based on historical data. In the automotive industry, this could involve predicting future sales based on economic indicators or forecasting vehicle reliability based on component failure rates.
- Cohort analysis involves grouping individuals or entities into cohorts based on shared characteristics and observing them over time. This could be used to track the performance and maintenance needs of vehicles from different production batches or model years.
- Variance analysis is used to understand the dispersion in a dataset. It’s crucial for quality control in manufacturing processes, helping identify when a process is deviating from the norm and pinpointing potential causes.
Experimental Design
Experimental design refers to planning experiments to systematically vary inputs or conditions so that reliable conclusions can be drawn about how these variations affect outcomes. In the automotive industry, this might be applied in (a few examples):
- Material testing: Assessing how different materials affect vehicle durability, safety, and performance.
- Engine design: Experimenting with different configurations to optimize fuel efficiency, power, and emissions.
A/B Testing
A/B testing, a specific type of experimental design, involves comparing two versions (A and B) to determine which one performs better on a given metric. In the automotive context, A/B testing could be used to:
- Evaluate different vehicle features with consumers, such as infotainment system layouts or driver assistance technologies.
- Test marketing strategies or promotions/campaigns to identify which approaches are more effective in attracting potential buyers.
Statistical Classification
Statistical classification involves categorizing entities into groups based on their features. In the automotive sector, this could be applied to:
- Fault diagnosis: Classifying vehicles or components as faulty or not based on diagnostic data.
- Customer targeting: Using customer data to classify buyers into “segments” more likely to be interested in specific vehicle models or features.
By applying these statistical concepts, the automotive industry can gain valuable insights into manufacturing processes, customer preferences, vehicle performance, and market trends. This not only helps in improving product quality and operational efficiency but also in designing strategies that cater to the dynamic needs and preferences of consumers.
In our next blog as part of this series, we’ll be focusing on the topic of “Optimization using ML and Data Science”.
More Publications
-
The Future of Payment Infrastructure: Overcoming Challenges & Embracing Innovation
-
The Current State of the Financial Services Industry: Key Challenges & Priorities for the Future
-
The Current State of Credit Unions: Challenges, Trends, and Solutions for Sustainable Growth
-
The Current State of Banking: Trends, Challenges, and Competitive Pressures